- Blog
- AI Across Industries
- AI for Real Estate Investment
AI for Real Estate Investment
How do you determine which real estate investment decision is better than another? Many real estate players have long made decisions based on intuition coupled with analyzing traditional key retrospective data. That traditional approach normally included analyzing how real estate property assets performed in the past but also often looking for market trends.
The million-dollar question when analyzing potential real estate investment decisions was, “Are your hypotheses based on intuition and your knowledge of the market, or are they based on hard evidence-based facts and data?”
Today, the use of AI in Real Estate is providing one of the most significant disruptors in the sector, catalyzing the connection between investors and firms, tenants and property managers, brokers, and buyers, regardless of location and time. Thanks to the increasingly rapid evolution of AI and advances in machine learning, the real estate industry has a more vivid picture of future risk and opportunities across all different market segments: offices, residential, retail, logistics, hotels, OPRE and data centers. Despite the great outcomes the use of AI in Real Estate promises, there are still some hurdles to overcome.
Unleash the Power of AI
Investors, asset managers, and developers often claim that one of the biggest challenges in real estate is the disconnection between the available data and the complexity of harnessing it for quick, actionable insights.
Investors and developers need to understand where to acquire real estate assets and when to trigger development, while portfolio managers need to optimize their holdings and recurrently evaluate real estate conditions to decide if they should divest or not. Being slower to identify and understand subtle and complex market trends translates to missed business opportunities, while being the first player who identifies the best opportunities in the market leads to significant economic rewards.
Next to this ongoing challenge of compiling and interpreting data, real estate players are also dealing with the challenge that real estate is increasingly driven by human needs and market shifts. Recent examples include the pandemic shock, which led to a temporary market freeze, followed by a demand and supply imbalance that brought to unexpected rises in real estate prices.
Why is it so hard to accurately predict real estate market changes? How can real estate investors, asset managers and developers keep track and preprocess massive fragmented data and quickly find key patterns to make profitable investments? And what has blocked them from doing so?
Traditional analytical approaches and data sources hamper the decision-making process to draw clear assumptions on market trends and develop robust and comprehensive business cases. Real estate investment analysts must review massive, fragmented data to identify clear historical patterns and place their judgements with little or non-automated supporting tools to get valuable insights from that material. In addition, traditional analytical approaches are not flexible enough in generating alternative scenarios that allow investment analysts to test market shifts or to compare predictive-model outputs to the forecasts of traditional sources of market information. By the time a real estate asset manager or investment analyst can collect and process the data needed to build an analytical approach, the best investment opportunities have vanished.
When AI Meets the Art of Possible
One way to bring together data through advanced analytics is to use an end-to-end enterprise AI platform, which makes it significantly easier to aggregate, interpret, and get valuable insights from massive and fragmented sources of data. End-to-end enterprise AI platform solutions automate the data preprocessing workflow, as well as the pipeline of building, training, deploying, and managing AI and machine learning models. Also, they can scale the AI lifecycle at a fraction of the cost compared to in-house alternative analytical solutions. At the end of day, it is not the raw data that creates value but the ability to extract quick and meaningful patterns and forecasts. These predictions then can be used to build and develop new real estate market strategies.
Real estate developers aim to identify underused but high-value land for development. AI and machine learning models can quickly identify areas of interest, then evaluate the potential of a given land from using a predictive viewpoint. Hence, a developer can immediately assess local data, coupled with market forecasts, and select the most relevant districts, neighborhoods, and types of buildings for development. Of course, that developer can also optimize product mix, price segmentation, and development timing to maximize the Net Asset Value and Net Operating Income as required.
Likewise, for an asset manager who aims to expand and optimize real estate portfolios, machine learning models can rapidly combine macro and local forecasts to target cities, districts, and neighborhoods with the highest demand and potential price and rental growth. This will enable the asset manager to detect real estate assets in areas that are undervalued but rising in key real estate market’s performance metrics.
An end-to-end AI analytical approach can also rapidly yield powerful inputs that inform new assumptions, challenge traditional intuition and judgments of market changes, as well as removing noise to identify what matters most. End-to-end AI enterprise solutions allow real estate investment players to have full flexibility to rapidly generate insights aimed at testing different potential situations and scenarios.
Examples may include: 1) testing expectations for submarkets or areas within high-growth markets, aiming to select properties to invest in or places to divest, 2) identifying submarkets or areas that will hold their value in otherwise declining markets, 3) comparing model outputs with alternative forecasts provided by traditional sources of the real estate market information (e.g., international organizations, brokers and advisory firms) and 4) identifying capital expenditure decisions at the real estate asset level; for example, calculating the yield and return on investments.
Forecasting the Real Estate Market Using DataRobot
As we discussed, the use of a successful end-to-end enterprise AI solution can yield powerful insights. DataRobot AI platform can be used to accurately forecast future real estate market changes at scale, while enabling real estate players to obtain quick and actionable insights for the business.
Asset managers and investors will be able to leverage DataRobot’s Automated Time Series capabilities to prepare and combine traditional and non-traditional data in order to build robust machine learning models to forecast real estate market fundamentals. For example, forecasting rents and prices at different levels of aggregation (e.g., market and submarket levels).
Another major advantage in using DataRobot’s platform for real estate players is that they will be able to build no-code based AI Applications. This will empower real estate players to build their own AI applications to be scaled for use in other scenarios, thus unblocking them from not obtaining quick insights on real estate market conditions, while helping them to be the first mover in real estate investment decisions. For instance, DataRobot’s AI applications can be used to visualize and analyze the forecast of real estate rents and prices, but also it can be used to build alternative forecast scenarios (i.e., what-if scenarios).
Last but not least, DataRobot’s AI platform capabilities help real estate investors and asset managers to be prepared for instant real estate market shifts or unexpected events, thereby allowing them to obtain accurate and reliable predictions at any time to mitigate risk to its business. For example, DataRobot MLOPS with Continuous AI automatically maintains all production models, keeping them at peak performance regardless of real-world unexpected events and scenarios. This is highly useful for the real estate players, as unexpected events like the COVID-19 pandemic or, more recently, the supply-chain crisis had a significant impact on the real estate market and proved to all of us that data-driven approaches can be at risk in an instant.
Now, let’s take a closer look at what an end-to-end, data-driven approach to forecast real estate market changes with DataRobot AI platform looks like.
DataRobot Time Series Modeling
DataRobot’s Automated Time Series Modeling rapidly builds forecasting models. Time series modeling is different from other types of machine learning and requires specialized data handling and preprocessing, as well as modeling techniques.
DataRobot automatically identifies the unit of interest to forecast (e.g., districts) as different series in the dataset and treats them independently. DataRobot also allows users to automatically cluster (e.g., center city, prime suburban and suburban cities) these different series and perform time series segmented modeling, leading to improved model performance and decreased time to deployment. DataRobot also automatically handles complicated time series requirements like data and time partitioning, as well as feature engineering processes aimed to construct time series features based on the characteristics of the data.
On top of that, using DataRobot’s built-in automation workflow, either through the no-code Graphical User Interface (GUI) or the code-centric DataRobot Core, users have access to the full-spectrum of time-based machine learning models, while generating explainable predictions and visualizations, which increases model explainability and builds trust with users.
Forecasting Prime House Prices
In this example, DataRobot AI Platform is used to forecast the next year’s house price at each submarket (e.g., district or neighborhood level), clustered into different markets (e.g., city level). From the modeling training dataset, we can observe the target and the input features. Input features include macroeconomic, sociodemographic and real estate market’s supply-demand variables. The data is available at quarter intervals. We will use the “Date” column to set up a time-aware modeling. This will allow us to leverage the DataRobot’s Automated Time Series models.
We will build models to forecast Prime House Prices at the district level for the next year. Users can decide to use, if necesary, segmented modeling to improve scalability and model segment-dependent behaviors and patterns (e.g., across different cities). Also, known in advance variables can be used to define those variables for which you may know the future value in advance. This is very useful when users would like to either use macroeconomic forecasts provided by traditional sources (e.g., FED, ECB and OECD) or running what-if scenarios to benchmark their own scenario’s model outputs against alternative forecasts provided by external sources (e.g., advisory firms and brokers). All of these options can be seen in the following project settings.
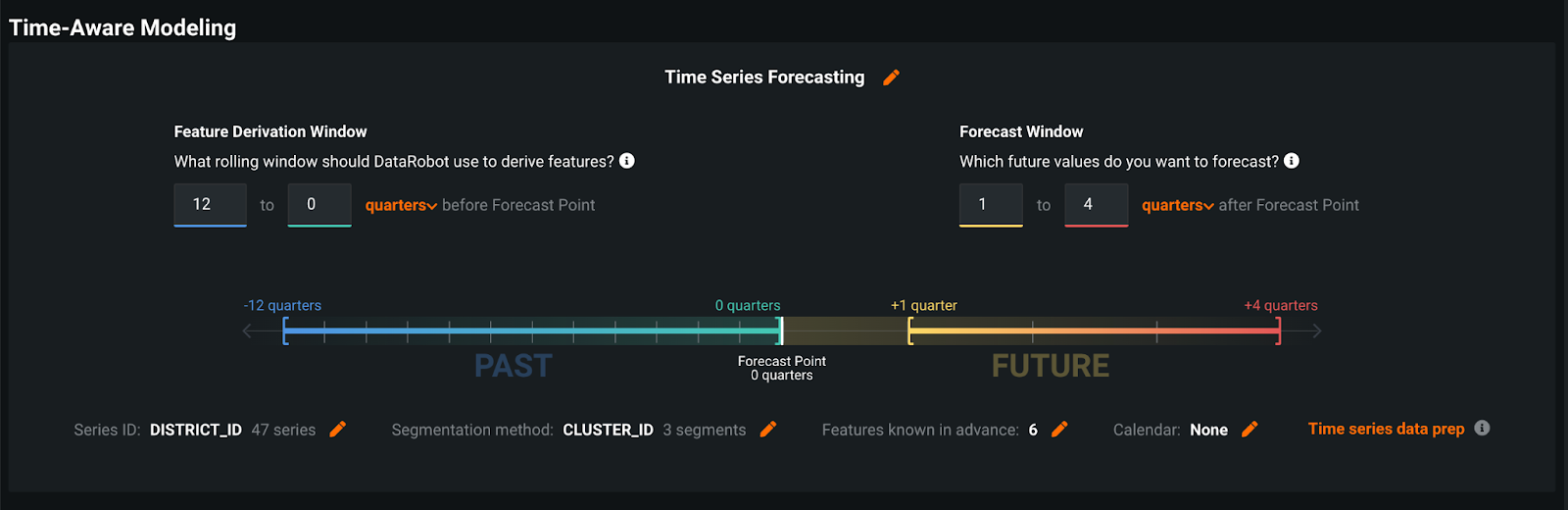
A 12-quarter feature derivation window generated the best results for forecasting the prime house prices of the next year. DataRobot automatically determines the best backtest configuration for the dataset. However, we can customize it further.
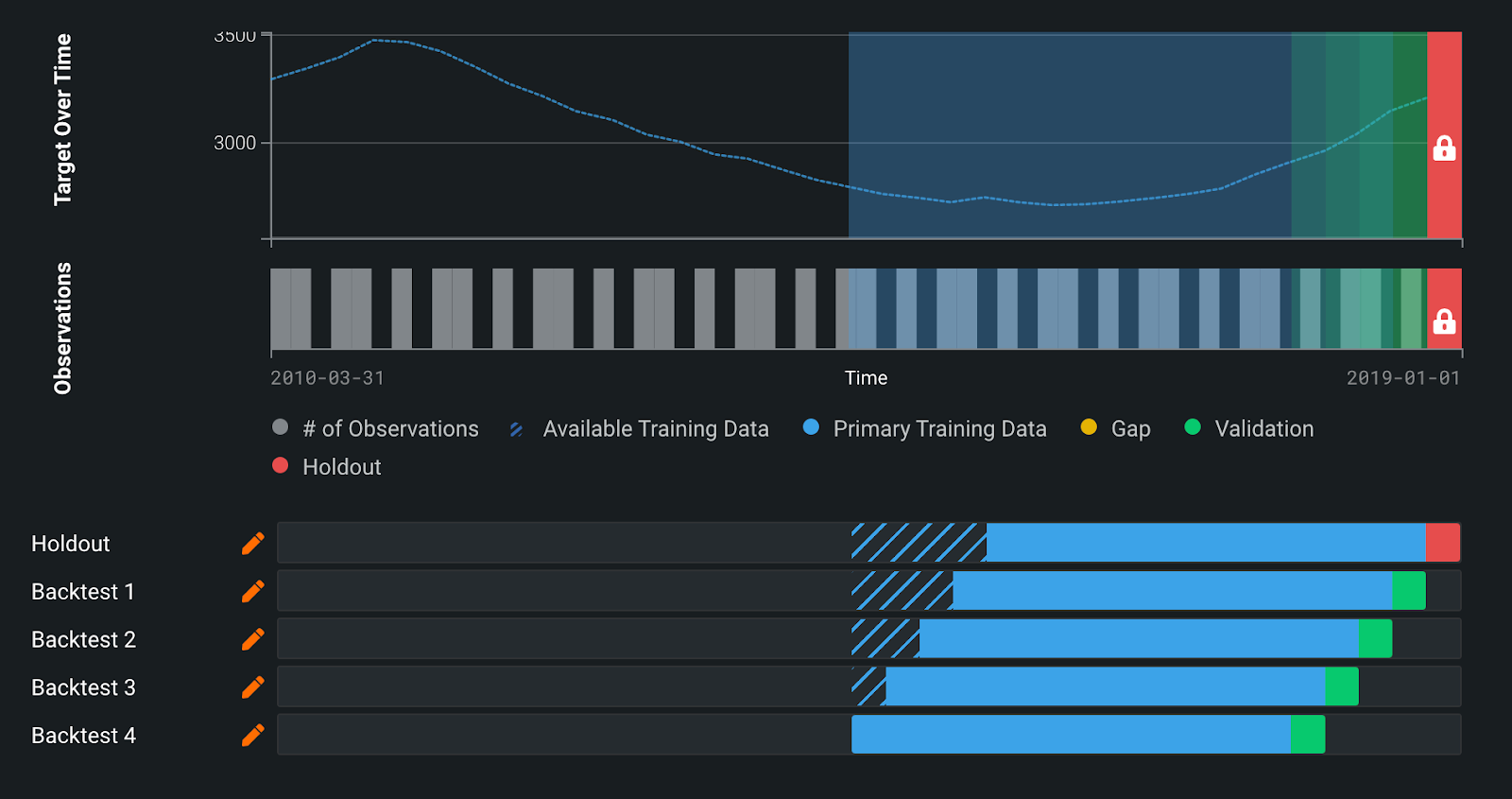
DataRobot starts modeling after we enable some additional settings like including advanced ensembling and blueprints, as well as cross-series feature generation, to leverage information across multiple series, potentially yielding insight into recent overall market trends.
Explore Model’s Performance and Get Quick Insights
DataRobot also took the 22 original input features and automatically generated +250 new features and a distinct set for each different segment (i.e., center city, prime suburban and suburban cities) during the feature engineering process to increase the model performance. Using these new time-aware features, DataRobot automatically built more than 40 models for each different segment for comparison. For example, DataRobot had generated for the suburban cities segment 255 time-aware features from the 22 input features and determined that 145 features were enough for an accurate and fast model.
The recommended model shows a good accuracy over time and is very reasonably stable across backtests and holdout fold.
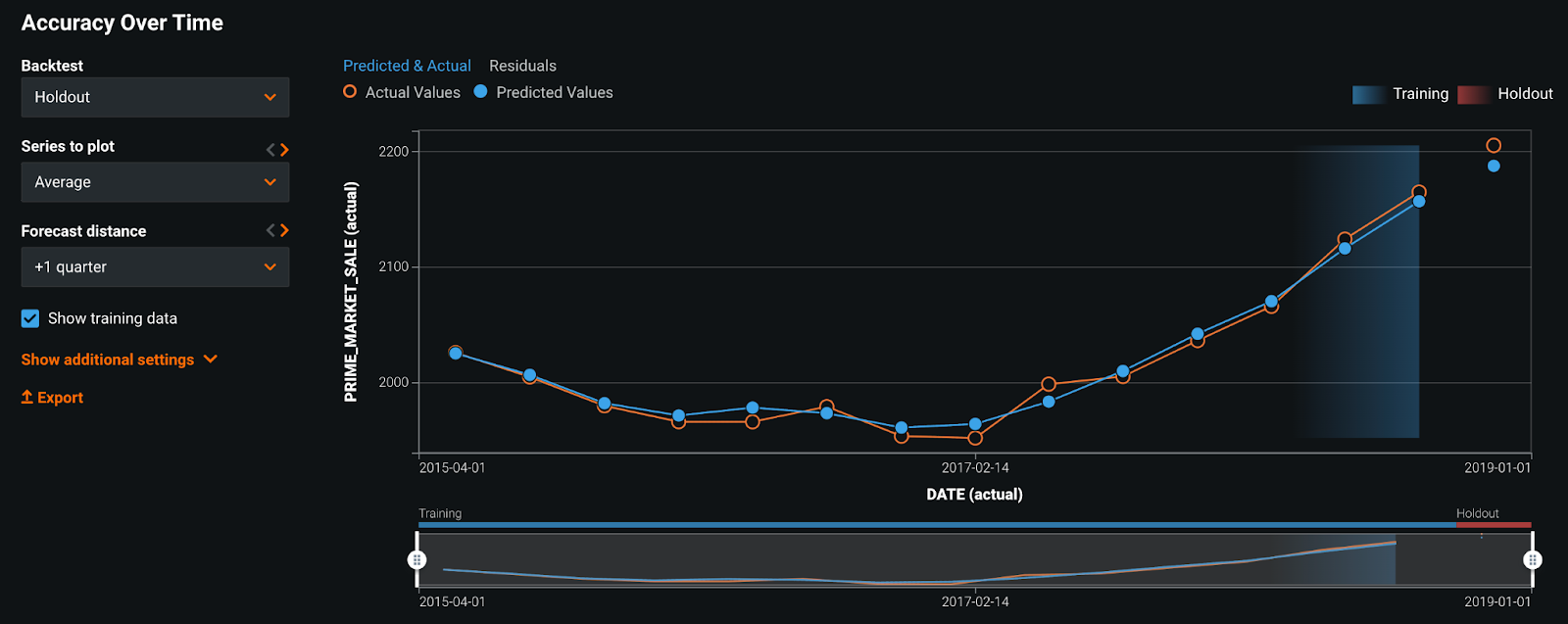
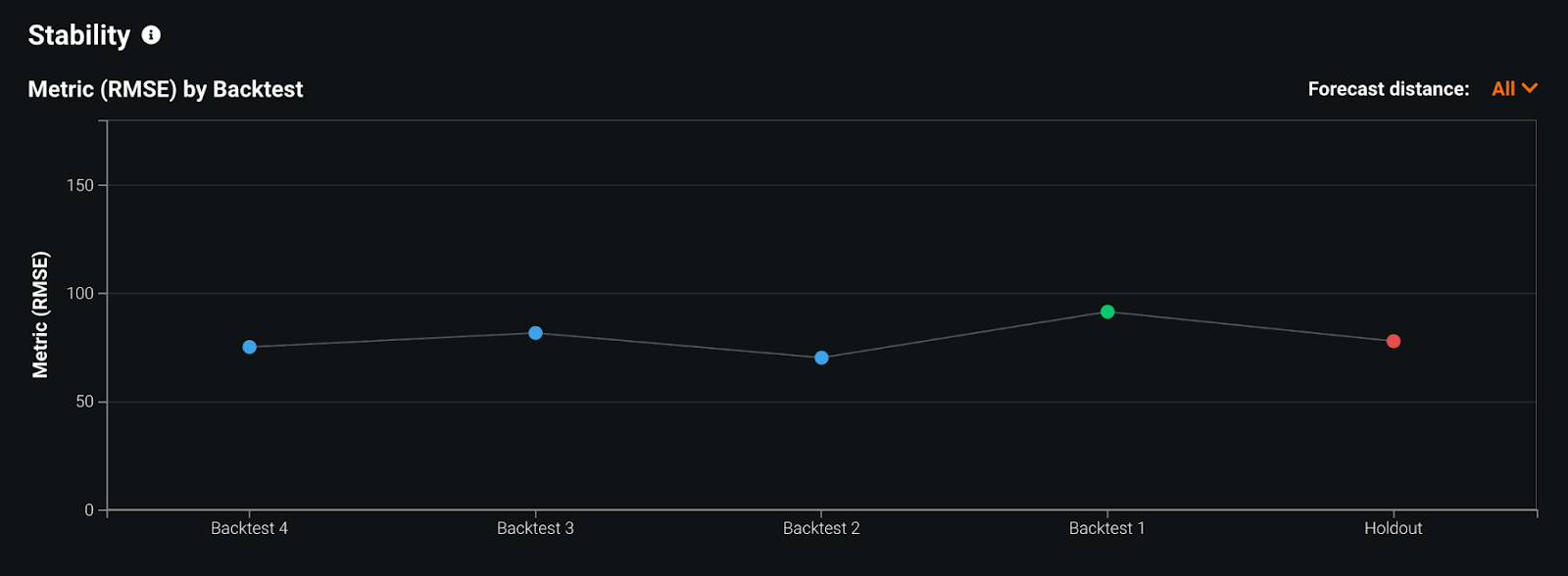
Looking at DataRobot’s Explainable AI capabilities like feature impact among others (feature effects and prediction explanations), real estate players will be able to understand how machine learning models reached their findings by making AI technology as transparent as possible. With regard to feature impact capability, users can disentangle what factors determine the prime house price. For instance, we can observe key drivers of forecasting house prices in the prime suburban market segment below.
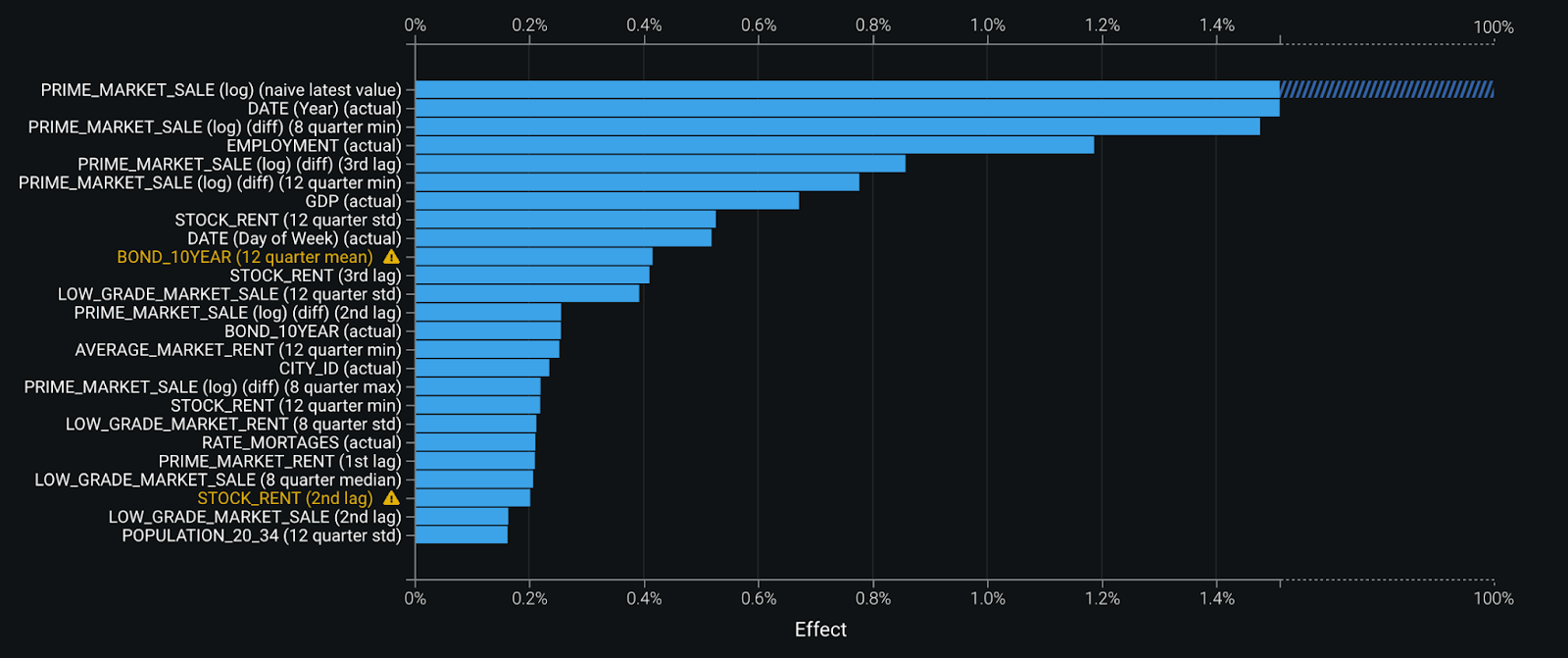
DataRobot AI Applications
By bringing the recommended time series model to DataRobot’s AI Apps, real estate investors and asset managers can easily get intelligent AI Applications that are predictive to changing market conditions and adaptive to an ever-changing environment.
Within the AI App, real estate players can compare real estate market change forecasts (e.g., prime house prices) with actual values, provide insights on prediction explanations over time, and dig deeper into the reasons driving each forecast.
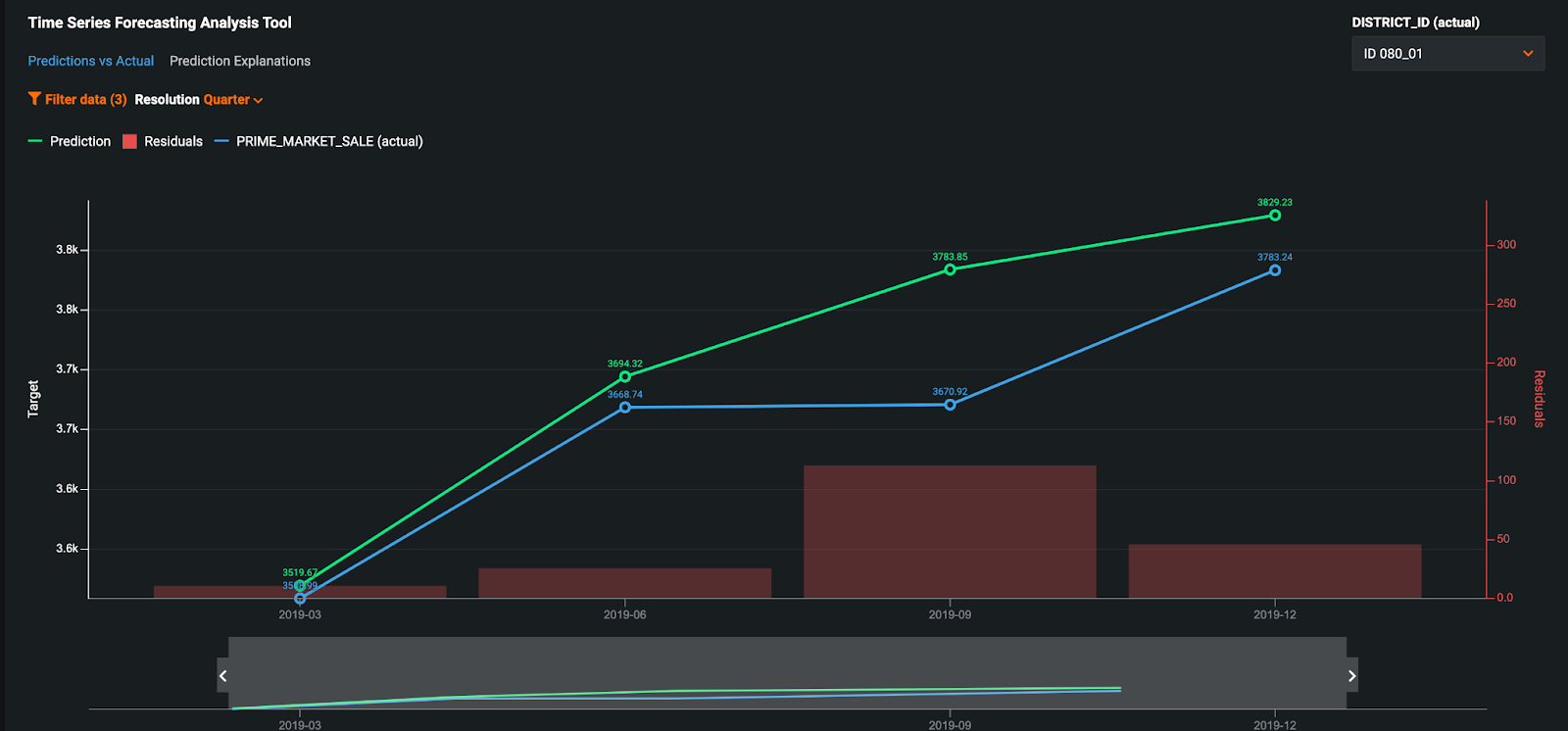
Interestingly, investors and real estate managers can also quickly create their own scenarios based on their intuition and knowledge of the market, or introduce alternative forecast scenarios provided by traditional sources (e.g., international organizations and advisory firms) to benchmark model outputs based on different assumptions on both macroeconomic and real estate local market trends.
For example, real estate investment analysts will be able to add new scenarios for each real estate submarket or market by changing both macroeconomic (e.g., GDP, CPI and construction costs) and local (e.g., city’s employment and district’s population aged between 20-34) factors. DataRobot will rapidly generate new insights aimed at helping real estate players to have full flexibility in testing different potential situations and scenarios as we can see below.
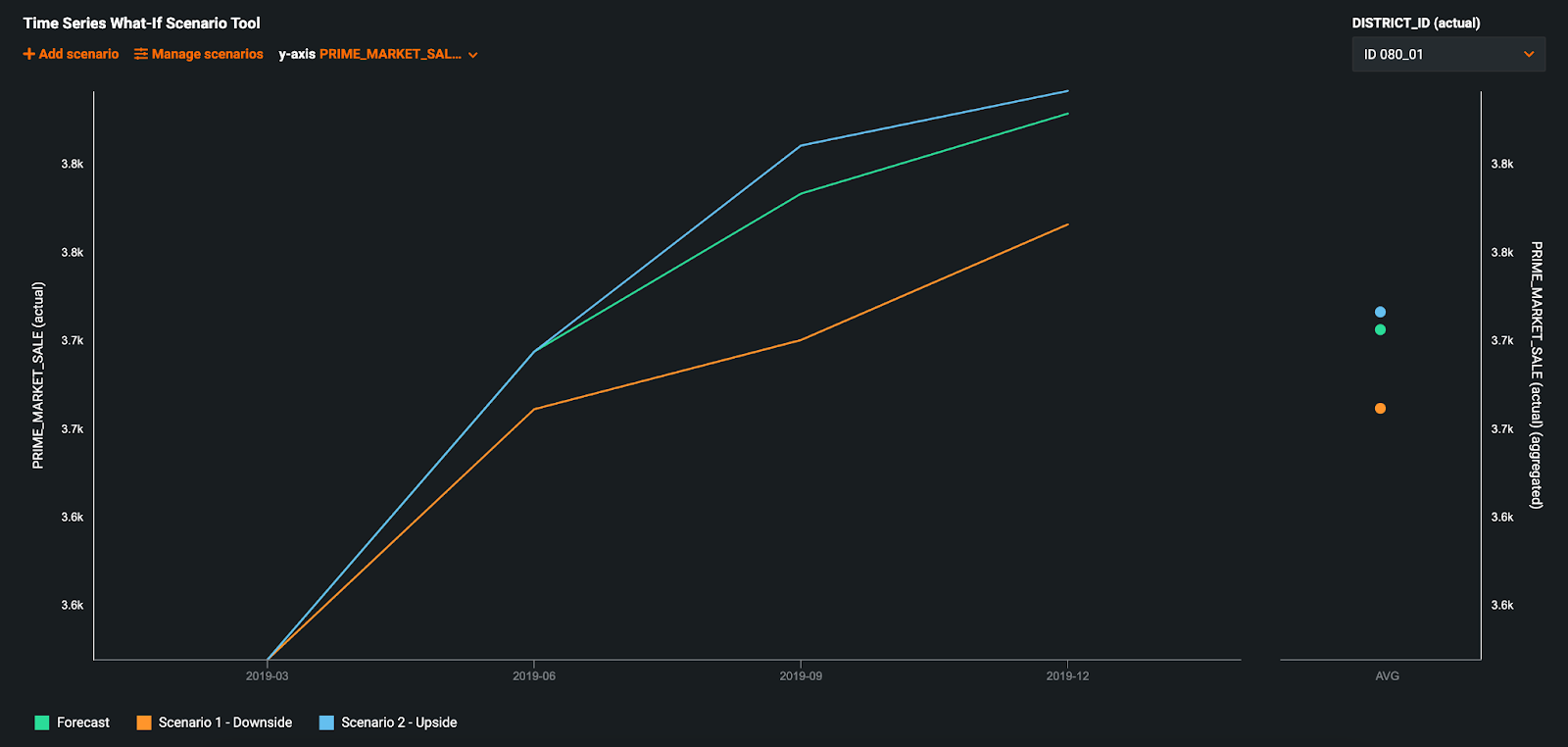
With AI Applications, DataRobot definitely empowers real estate asset managers and investors to be the first movers in a highly competitive market segment, maximizing the return on investments by identifying the best investment opportunities, while mitigating the potential risk related to any market change shift and unexpected events or trends.
Conclusions
The DataRobot platform is suitable for all user personas—from advanced data scientists and data analysts to business leaders and cross-functional teams—empowering everyone to solve all types of business problems and challenges by democratizing AI.
We have just shown how DataRobot quickly produced a multi-series time series with segmented modeling forecasting model to accurately predict real estate market changes, while ensuring real estate players can successfully overcome all existing challenges regarding the real estate investment business.
DataRobot AI Platform is able to analyze patterns and make predictions based on the data that’s being analyzed. However, predictions for improving the quality of the potential investment’s future performance can be extensive. They include not only an analysis of performance at the market and submarket levels like forecasting real estate market changes but also an analysis of performance at the property level like tenant patterns and predictive maintenance. DataRobot’s platform can also be applied to numerous use cases at the real estate property level aimed at optimizing both the Net Asset Value (NAV) and the Net Operating Income (NOI). Examples may include predicting asset’s value and time-to-sell for massive real estate portfolios and predicting which tenants will renew and which ones will leave the property when their lease expires, thereby helping to maintain a higher occupancy rate.
Contact a member of the DataRobot team to learn more and see how your organization can become AI-driven.
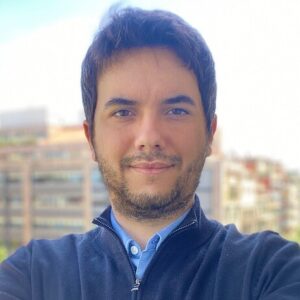
Senior Customer-Facing Data Scientist, Team Lead at DataRobot
Jaume is a Senior Customer-Facing Data Scientist, Team Lead at DataRobot. He leverages the DataRobot AI Platform to drive the adoption of AI and machine learning in major enterprises in EMEA, with a specific focus within the Banking, Insurance, and Utilities Industries. Prior to DataRobot, Jaume worked in a variety of data-centric roles in universities and major enterprises for more than 10 years, both as an individual contributor and director of data science. During this time period, he had the opportunity to build and lead many analytics products and data advisory services, with a special focus on the Real Estate sector, leveraging machine learning and applied statistics.
Jaume holds a PhD. in Statistics and Operations Research from UPC-BarcelonaTech and a PhD in Urban and Regional Economics from TU Delft and in his spare time enjoys traveling and playing chess.
-
Improving cash flow: The AI advantage in financial forecasting
March 5, 2025· 4 min read -
What misbehaving AI can cost you
February 26, 2025· 9 min read -
How to use DeepSeek-R1 for enterprise-ready AI
February 18, 2025· 7 min read
Latest posts